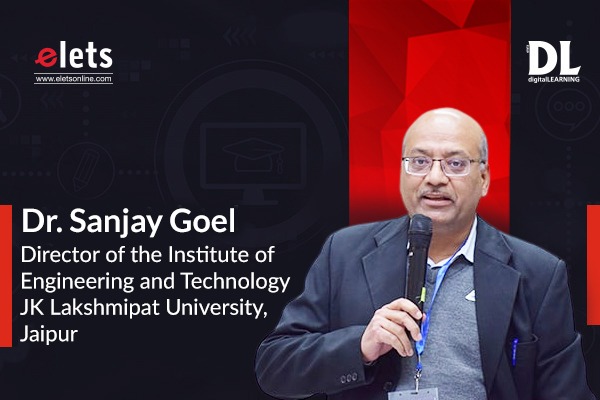
“Digital humanities” is an interdisciplinary field that combines the methodologies and insights of the humanities, such as literature, history, and philosophy, with digital technologies and computational methods. It aims to transform the ways in which scholars research, analyse, and interpret human culture and historical records by leveraging the power of digital tools and computational techniques. In digital humanities, researchers use various tools and methods like text mining, natural language processing (NLP), data visualization, network analysis, geographic information systems (GIS), etc., to study and explore large datasets, digitized texts, and multimedia resources. This enables them to uncover new patterns, connections, and insights that would be difficult to identify using traditional research methods.
Natural Language Processing (NLP) involves various computational techniques and methods for understanding, interpreting, and generating human language. Text mining, a subset of NLP, focuses on extracting patterns, trends, relationships, and valuable insights. The roots of text mining can be traced back to the late 20th century when computational linguistics and information retrieval began to intersect with research in humanities and social sciences. Early pioneers utilised simple keyword searches and frequency analysis to identify key themes in large corpora. Over time, with the development of more sophisticated algorithms and computational tools, text mining and NLP have become indispensable tools for understanding the previously unexplored complexities of human culture and history.
One of the most significant applications of text mining in historical studies is the analysis of large corpora of historical texts. For example, researchers use text mining to analyse historic newspapers archived by the Chronicling America project of the United States from 1770 to 1963, enabling them to identify trends and patterns in news reporting, advertising, and public opinion during different periods. Similarly, researchers use the Old Bailey Proceedings Online corpus of nearly 200,000 trials from the Old Bailey, London’s central criminal court, spanning the period from 1674 to 1913, to identify trends in crime, punishment, and social attitudes over time. It can be employed to study the linguistic features of historical documents, such as grammar, syntax, and vocabulary. This can reveal interesting insights into language evolution and help trace the origin and dissemination of specific linguistic features across time and space. For example, the Helsinki Corpus of Historical English Texts project uses text mining to analyse the linguistic features of English texts from the Old English period to the early Modern English period.
Topic modelling, a text-mining technique that identifies recurring topics and themes within a corpus of documents, has proven invaluable in historical research. By applying this technique, researchers can identify patterns and trends in large collections of historical texts, such as newspapers, legal documents, and personal correspondence. For example, researchers applied topic modelling to analyse the content of the “Richmond Daily Dispatch,” a newspaper published during the American Civil War, to analyse the newspaper’s coverage of events, issues, and public opinion.
Text mining enables researchers to analyse the literature and explore patterns in style, themes, and influences across various time periods and cultural contexts. For example, the Culturomics project at Harvard University discovers cultural trends using text mining on a massive corpus of digitised books. By tracking the usage of words and phrases over time, the researchers can analyse how cultural ideas have evolved and spread. In another example, the Stanford Literary Lab applies text mining to analyse the themes and narrative structures of novels. Computational Stylistics has emerged as a field of enquiry that examines the forms, social embedding, and aesthetic potential of literary texts by means of computational and statistical methods. It is used to investigate literary texts for a variety of research questions, including authorship attribution, style, genre, and epoch; literary topoi, plot, and character networks; narrative perspective, figure characterization, and emotion; gender, race, and social status; canonicity, literariness, and textual quality; and cognitive representations of the word beauty, metaphor, and rhyme.
Text mining of literature and other textual sources has been widely used by researchers, providing valuable insights on various other issues in the past. For instance, exploring gender and race in historical texts helps identify patterns and trends in the portrayal of marginalized groups, shedding light on societal attitudes and biases. Similarly, sentiment analysis in historical texts allows researchers to understand the emotions and attitudes expressed by authors during specific time periods or events, giving insights into their experiences and perceptions. It has been applied to analyse artistic and architectural descriptions, historical speeches and debates, religious texts and beliefs, historical medical texts, and propaganda and media coverage. By examining the language, themes, and characterizations in these texts, researchers can identify patterns and trends in artistic and architectural styles, understand the rhetoric and priorities of historical figures, trace the evolution of religious thought and medical knowledge, and assess the role of media in shaping public opinion.
Text mining has also been applied to study the social networks of historical figures and events by extracting information from historical records, letters, and diaries, to map out connections between individuals and groups, shedding light on the dynamics of historical events. For example, the Mapping the Republic of Letters project at Stanford University uses text mining techniques to analyse the correspondence networks and other records of early modern scholars. In another example, researchers at Carnegie Mellon University and Georgetown University have developed “Six Degrees of Francis Bacon,” an innovative digital humanities project that reconstructs the social network of early modern Britain. This project website allows users to explore the personal connections between prominent figures such as Francis Bacon, William Shakespeare, Isaac Newton, and many others. At present, “Six Degrees of Francis Bacon” features over 13,000 individuals and approximately 200,000 relationships, offering a unique perspective on the interconnectedness of key historical figures.
NLP has been applied to aid in the preservation of endangered languages and to broaden the accessibility of historical texts. Machine learning algorithms are now capable of translating texts between languages, connecting diverse cultures and time periods. Both the Rosetta and Perseus projects incorporate NLP techniques to improve the accessibility and comprehension of texts within their digital libraries. The Rosetta Project concentrates on creating a digital library of human languages, including endangered ones, using NLP for translation, categorization, and language pattern analysis. In contrast, the Perseus Digital Library offers translations and linguistic resources for classical texts from Greco-Roman antiquity, utilising NLP for language analysis, text annotation, and machine translation.
NLP has been instrumental in deciphering ancient scripts. By analysing patterns, character distributions, and potential language similarities, it can help reconstruct lost languages and decipher ancient texts. For example, the decipherment of the ancient Hittite language was assisted using NLP techniques, which enabled the researchers to identify word patterns and grammatical structures. This breakthrough allowed for the translation of numerous Hittite inscriptions.
NLP has been applied to the analysis of oral histories, which provide invaluable insights into the lived experiences of individuals and communities in the past. By transcribing, annotating, and analysing recorded interviews, NLP can help identify patterns, themes, and connections in these narratives. For example, NLP has been applied to transcribe, index, and analyse the testimonies archived in the Shoah Foundation’s Visual History Archive, which contains around 55,000 video testimonies of Holocaust survivors.
Conclusion:
In conclusion, Natural Language Processing and its subset, text mining, have revolutionized the study of human culture and history. By extracting patterns, insights, and valuable information from vast volumes of textual data, researchers can now explore previously inaccessible dimensions of the past. The continuous development of NLP and text mining techniques will open new possibilities and enhance our understanding of human history and culture through interdisciplinary collaboration between IT, humanities, social sciences, and cultural studies. To prepare students to participate in such work, higher education institutions must foster an environment that encourages interdisciplinary learning, integrating computational techniques and methodologies with traditional humanities and social science curricula. By equipping students with the necessary skills and knowledge, universities can play a vital role in shaping the future of research in these fields, facilitating innovative discoveries through the power of NLP and text mining. Computer science students and faculty have a great opportunity to collaborate with their counterparts in humanities and cultural studies departments to apply NLP and text mining technologies in their domain.
Views expressed by Dr. Sanjay Goel, Director (Institute of Engineering and Technology), JK Lakshmipat University, Jaipur, Rajasthan
