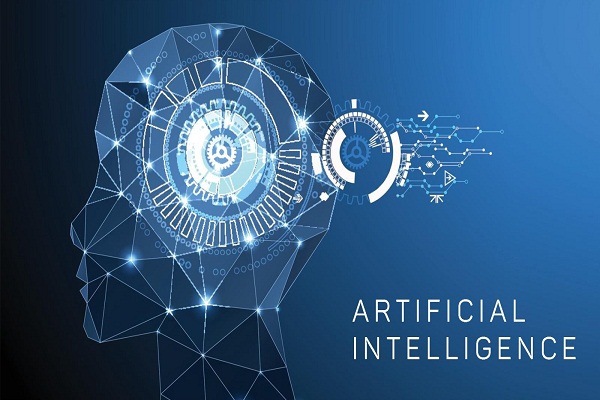
Machine learning and big data have afforded tremendous improvements to almost every field, including higher education. For example:
- The publisher Elsevier is using AI to analyze literature reviews, measure plagiarism, and identify forged numerical or statistical features and details. This will ensure that unethical behavior is flagged before any publication goes live.
- Intelligent chatbots based on natural language programming (NLP) are being already used by universities across the globe. The Technical University of Berlin (TUB), for example, has developed a chatbot system that can guide students around campus and help them choose their courses. Administrators at Spain’s University of Murcia were surprised to learn that its chatbot system answered 91% of 38,708 questions accurately. The chatbot enabled the university to operate outside of working hours and had a positive psychological impact on students—they became more motivated to use the chatbot over time, knowing there would be a tool to communicate directly with the university administration on an ongoing basis.
- Virtual assistants play a key role at many universities. Carnegie Mellon University, through its Open Learning Initiative (OLI), has developed AI-induced cognitive tutors to engage students. This had positive results in students’ overall performance and dedication levels. Similarly, at Georgia Institute of Technology, a virtual teaching assistant (TA), using IBM’s Watson Platform, was implemented in order to provide responses to about 40,000 questions during the course ‘Knowledge-Based Artificial Intelligence’. This ensured the prevention of low student retention rates and positive class engagement.
What AI Can Bring to Higher Ed
A New Way to Plan Programs
If we consider curriculum development, AI’s speed, accuracy, and consistency can ensure that an adequate subject selection and distribution will be established based on pre-set parameters by the educator or administrator. This would enhance the institution’s dynamic adaptation to the growing number of students and new programs.
Bias-Free Admissions Management
Machine learning utilizes the incommensurable power of big data to expand the number of options and scenarios of any complex planning in an institution, such as admissions management.
Large-Scale Learning Analysis
The long-term benefits of implementing learning analytics in higher education can be:
- Improving student retention. For example, at the University of New England, the student attrition was reduced to 12% and the students displayed a growing sense of belonging to the class and learning community in general.
- Supporting informed decision making. In this case, at the University of Adelaide, educators were able to enhance the design of collaborative activities in based on the data collected. Additionally, learning analysis can provide inputs about the most suitable teaching assistants to be assigned to a particular group of students, as it is applied at the University of Edinburgh.
Scenario Planning and Improved Decision Making
Machine learning automation permits higher education administrators to scout tentative future scenarios by simulating alternative realities at a low cost and without the risks involved in real-life trial and error.
Implementing the Technology: Recommendations
The best way toward a full-fledged implementation is to provide an adjustment time frame period crossing the required stages upon this technological revolution:
- Implementation: the institution must define its own technological requirements depending on the work methodology, structure, program distribution, and financial investment required.
- Rejection: confusion and distress will take place as it is required to address the fact that these new technologies will take away several job roles and create unemployment at a certain rate.
- Adjustment: upon the regular use of this technology, the staff and students will understand the need for implementation and will show interest in understanding the mechanisms involved and long-term impact in the institutional productivity.
- Acceptance: also known as the Technology Acceptance Model (TAM), which explains the acceptance of new technologies by users based on three key elements: the technological usefulness, easiness to operate, and attitudinal approach.
- Continuous Development: after accepting the need and interactivity of this technology, users will feel the need to take part in future advances that these systems can bring about, acknowledging the durable benefits provided.
Dr. Raul V. Rodriguez is an assistant professor of artificial intelligence, machine learning, and robotics at Woxsen School of Business, India. He is an author, columnist, and registered expert in artificial intelligence, intelligent systems, and multi-agent systems at the European Commission. He is also a nominee for the Forbes 30 Under 30 Europe 2020 list.

